Data-Driven Approach Identifies Promising CO2 Conversion Catalysts(英語版のみ)
A research team has developed a unified theoretical framework to better predict the performance of single-atom catalysts (SACs) for electrochemical carbon dioxide reduction (CO2RR). Their model incorporates both pH and interfacial electric field effects—two critical factors that have often been overlooked or oversimplified in conventional catalyst studies.
CO2RR is gaining attention as a potential solution for reducing greenhouse gas emissions by converting carbon dioxide into useful products under mild conditions. Among the various outputs, carbon monoxide (CO) is especially valuable due to its use in syngas mixtures and chemical synthesis. However, catalyst behavior during CO2RR is known to be highly sensitive to the surrounding electrolyte environment, particularly local pH levels.
To address this, the team created a microkinetic model that operates at the reversible hydrogen electrode (RHE) scale. It explicitly accounts for the dynamic influence of pH and electric fields on reaction intermediates. This model was combined with spin-polarized density functional theory (DFT-D3) calculations and data-driven screening to evaluate 101 SAC configurations based on d-block transition metals.
The study examined SACs across a range of coordination environments, including pyrrole- and pyridine-functionalized graphene, covalent organic frameworks (COFs), porphyrins, and phthalocyanines. A consistent linear relationship was found between the adsorption energies of the key intermediates—∗COOH and ∗CO—indicating a predictable trend in binding behavior.
One of the notable findings of the study was the role of dipole–field interactions in modulating these intermediate bindings under varying pH conditions. These interactions helped explain why certain catalysts perform differently across pH environments, offering deeper insight into the mechanisms at play in CO2RR.
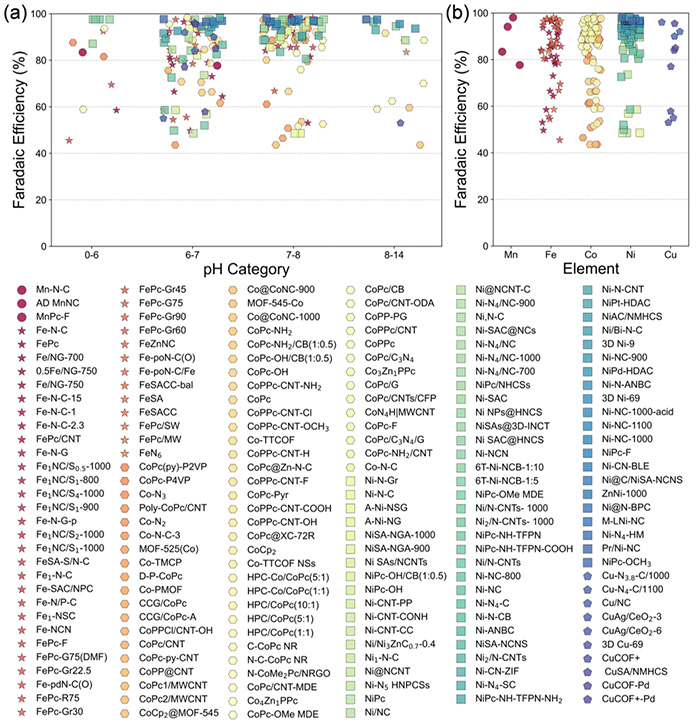
Activity analysis of 230 M–N–C catalysts for CO production through the CO2RR, compiled via large-scale data mining. ©Hao Li et al.
Model predictions showed strong agreement with available experimental turnover frequency data, suggesting the framework’s reliability in real-world applications. Based on this agreement, the researchers identified 12 SACs with favorable CO selectivity across a wide range of pH values. These catalysts are primarily centered around iron (Fe), copper (Cu), and nickel (Ni) atoms.
“This work represents a step toward more predictive catalyst screening by bridging theory with realistic electrochemical conditions,” said Hao Li, Professor at Tohoku University’s Advanced Institute for Materials Research (WPI-AIMR) and lead author of the study. “By integrating pH and field effects into our model, we can more accurately guide the design of catalysts suited for carbon-neutral energy technologies.”
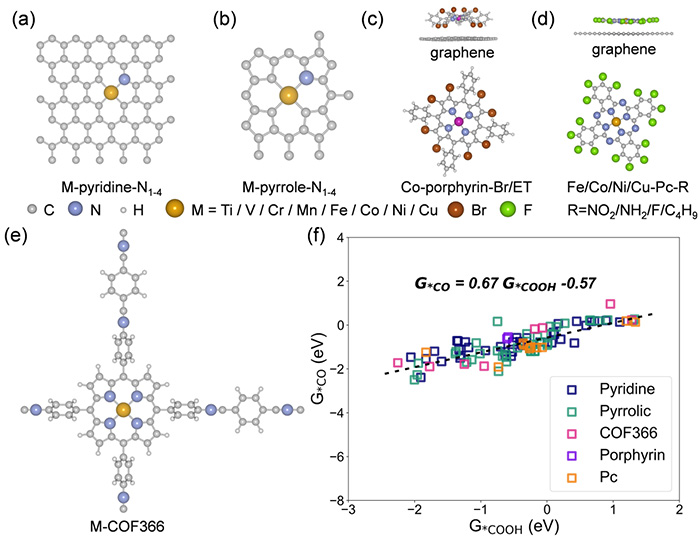
Representative M–N–C SAC structures considered in this study and the scaling relation observed in M–N–C SACs with various atomic configurations, where M represents the metal center (Ti/V/Cr/Mn/Fe/Co/Ni/Cu). ©Hao Li et al.
All computational structures used in the study have been uploaded to the Digital Catalysis Platform, a growing open-access resource for the catalysis community developed by Professor Li’s group. This contribution aims to support further research and data sharing across the field.
Looking ahead, the team plans to refine the model further to improve both predictive accuracy and computational efficiency. They also aim to incorporate machine learning algorithms to accelerate catalyst design and deepen understanding of pH-sensitive electrochemical reactions.
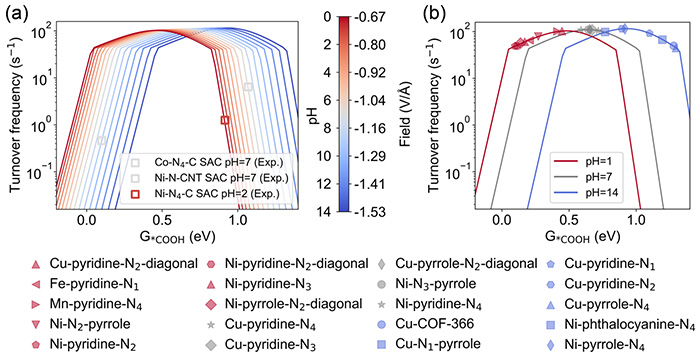
Analysis of the pH-dependent behavior and predictive modeling of CO2RR toward CO formation on d-block M–N–C SACs at −1.0 VRHE. ©Hao Li et al.
論文情報
タイトル: | Data-driven discovery of single-atom catalysts for CO2 reduction considering the pH-dependency at the reversible hydrogen electrode scale |
---|---|
著者: | Yue Chu, Yuhang Wang, Di Zhang, Xuedan Song, Chang Yu, and Hao Li |
掲載誌: | The Journal of Chemical Physics |
DOI: | 10.1063/5.0267969![]() |
問い合わせ先
研究に関すること
東北大学材料科学高等研究所(WPI-AIMR)
教授 Hao Li(研究者プロフィール)
E-mail: | li.hao.b8@tohoku.ac.jp |
---|---|
Webstie: | Hao Li Laboratory![]() |
報道に関すること
東北大学材料科学高等研究所(WPI-AIMR) 広報戦略室
Tel: | 022-217-6146 |
---|---|
E-mail: | aimr-outreach@grp.tohoku.ac.jp |