Benchmarking Theory with Experiments for Oxygen Reduction Catalysts
Tohoku University researchers have created a reliable means of predicting the performance of a new and promising type of catalysts. Their breakthrough will speed up the development of efficient catalysts for both alkaline and acidic environments, thereby saving time and effort in future endeavors to create better fuel cells.
Details of their research were published in the journal Chemical Science on March 15, 2024.
Fuel cell technology has long been heralded as a promising avenue for clean energy, but challenges in catalyst efficiency have hindered its widespread adoption.
Molecular metal-nitrogen-carbon (M-N-C) catalysts boast distinctive structural properties and excellent electrocatalytic performance, particularly for the oxygen reduction reaction (ORR) in fuel cells. They offer a cost-effective alternative to platinum based catalysts.
One such variant of M-N-C catalysts are metal-doped azaphthalocyanine (AzPc). These possess unique structural properties, characterized by long stretching functional groups. When these catalysts are placed on a carbon substrate, they take on three-dimensional shapes, much like a dancer placed onto a stage. This shape change influences how well they work for ORR at different pH levels.
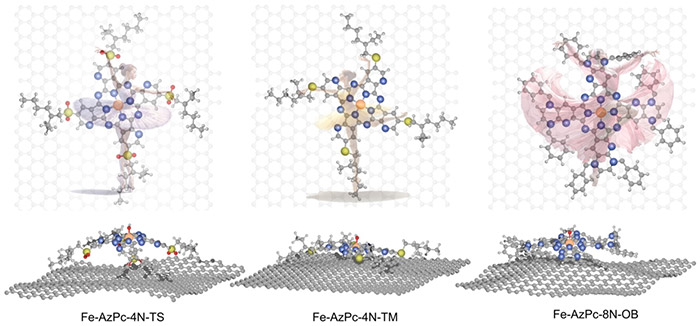
Structures of long-chain Fe-Azaphthalocyanines (AzPc) molecular catalysts. After DFT geometric relaxations with more than 650 atoms, different “dancing patterns” emerged due to the varying interactions between the molecular side chains and the graphene substrate. ©Hao Li, Hiroshi Yabu et al.
Still, translating these beneficial structural properties into increased performances is a challenge, one that requires significant modeling, validation, and experimentation, which is resource intensive.
“To overcome this, we used computer simulations to study how the performance of carbon-supported Fe-AzPcs catalyst for oxygen reduction reactions changes with different pH levels, by looking at how electric fields interact with the pH and the surrounding functional group,” says Hao Li, associate professor at Tohoku University's Advanced Institute for Materials Research (WPI-AIMR) and corresponding author of the paper.
In analyzing Fe-AzPcs performance in ORR, Li and his colleagues incorporated large molecular structures with complex long-chain arrangements, or ‘dancing patterns,’ with arrangements of over 650 atoms.
Crucially, the experimental data revealed that the pH-field coupled microkinetic modeling closely matched the observed ORR efficiency.
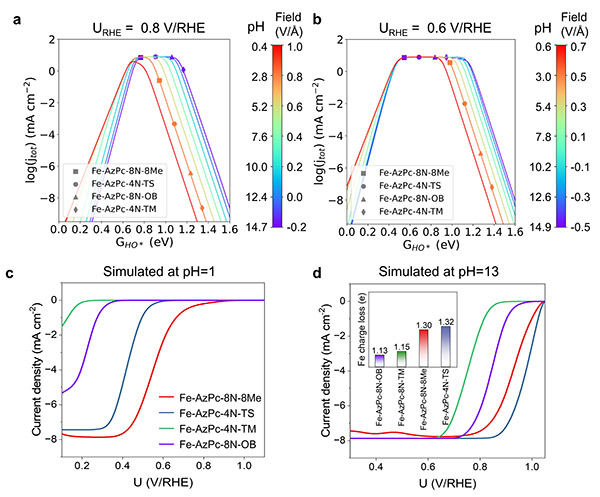
pH-dependent ORR volcano models and the simulated LSV curves of Fe-AzPc derivatives. pH-field dependent volcanos. The left and right sides of the color bar represent the correlation between the electric field and pH. This figure serves as a benchmark for our experiments. ©Hao Li, Hiroshi Yabu et al.
“Our findings suggest that evaluating the charge transfer occurring at the Fe-site, where the Fe atom usually loses approximately 1.3 electrons, could serve as a useful method for identifying suitable surrounding functional groups for ORR,” adds Li. “We have essentially created a direct benchmark analysis for the microkinetic model to identify effective M-N-C catalysts for ORR across various pH conditions.”
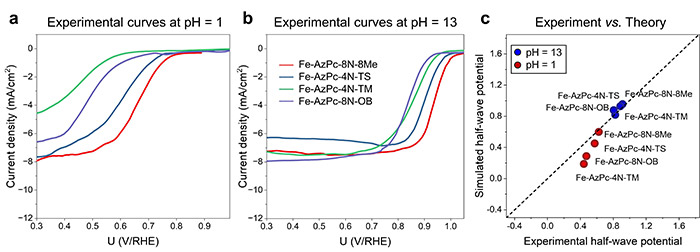
Experimental RDE polarization curves are provided at pH = 1 and pH = 13. This figure offers direct comparisons between the experimental and simulated half-wave potentials. ©Hao Li, Hiroshi Yabu et al.
Publication Details
Title: | Benchmarking pH-field coupled microkinetic modeling against oxygen reduction in large-scale Fe–azaphthalocyanine catalysts |
---|---|
Authors: | Di Zhang, Yutaro Hirai, Koki Nakamura, Koju Ito, Yasutaka Matsuo, Kosuke Ishibashi, Yusuke Hashimoto, Hiroshi Yabu*, and Hao Li* |
Journal: | Chemical Science |
DOI: | 10.1039/D4SC00473F![]() |
Contact
Hao Li
Advanced Institute for Materials Research (WPI-AIMR), Tohoku University
E-mail: | li.hao.b8@tohoku.ac.jp |
---|---|
Webstie: | Hao Li Laboratory![]() |
Hiroshi Yabu
Advanced Institute for Materials Research (WPI-AIMR), Tohoku University
E-mail: | hiroshi.yabu.d5@tohoku.ac.jp |
---|---|
Webstie: | Yabu Laboratory![]() |